
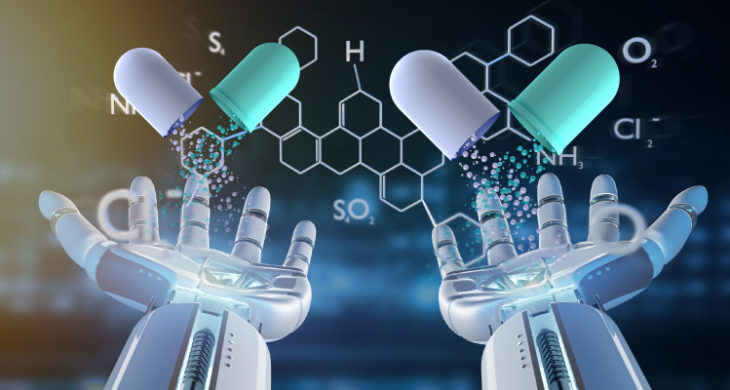
AI in drug discovery is an exciting development in healthcare. It integrates artificial intelligence to improve the process. The marriage of advanced algorithms, big data, and cutting-edge technology has unlocked new possibilities that have the potential to revolutionize the pharmaceutical industry. This article explores the profound impact of AI in drug discovery, shedding light on its capabilities, recent breakthroughs, and future prospects.
Drug discovery has traditionally been a painstakingly slow and costly process, often taking years and billions of dollars to bring a single drug to market. The typical approach involves identifying a molecule associated with a disease.
Lab tests are conducted to discover compounds that interact with this molecule. Subsequently, these compounds are tested to determine their effectiveness and safety. This process is not only time-consuming but also prone to high failure rates, leading to substantial financial losses.
The need for a more efficient and effective drug discovery process has driven researchers and pharmaceutical companies to explore innovative solutions. AI, with its ability to process vast amounts of data and identify patterns that might elude human scientists, has emerged as a game-changer in this field.
AI in drug discovery has gained momentum in recent years, thanks to advancements in machine learning, deep learning, and natural language processing. These technologies enable AI systems to analyze complex biological and chemical data, predict potential drug candidates, and even design novel molecules with specific properties.
One of the key advantages of AI is its ability to handle massive datasets. Researchers can feed AI algorithms with a wealth of information, including genetic data, clinical trial results, chemical structures, and scientific literature. AI systems can then sift through this information to identify potential drug targets and compounds more efficiently than traditional methods.
Furthermore, AI can accelerate the drug discovery process by predicting the safety and efficacy of drug candidates. Machine learning models can analyze historical data on drug development and outcomes to make informed predictions about the likelihood of success for a particular compound. This predictive power can significantly reduce the time and resources required for preclinical and clinical trials.
AI's impact on drug discovery is not just theoretical; it has already produced tangible results. Several notable breakthroughs showcase the potential of AI in this field:
AI algorithms have been used to identify existing drugs that could be repurposed for new medical conditions. By analyzing molecular data and disease pathways, AI can suggest potential candidates for further investigation. This approach has the advantage of significantly reducing the time and cost of drug development, as repurposed drugs have already undergone safety testing.
Identifying the right target molecule for a disease is a critical step in drug discovery. AI can analyze genomic and proteomic data to pinpoint potential targets with a high degree of precision. This targeted approach increases the likelihood of developing effective therapies.
AI-powered drug design is a cutting-edge application of machine learning. AI models can generate novel molecular structures with desired properties, optimizing drug candidates for specific biological targets. This has the potential to create entirely new classes of drugs.
AI can improve the efficiency of clinical trials by selecting suitable patient populations, predicting patient responses, and monitoring trial progress in real-time. This leads to faster recruitment, reduced costs, and more reliable results.
AI can analyze genetic and clinical data to tailor drug treatments to individual patients. This approach, known as precision medicine, maximizes therapeutic effectiveness while minimizing side effects.
Several real-world examples demonstrate the transformative power of AI in drug discovery:
In June 2023, a groundbreaking milestone was achieved when an AI-generated drug began clinical trials in human patients. Developed by a team of researchers, this drug was designed entirely by AI algorithms, showcasing the potential of AI to create novel drug candidates. While the outcome of the trials is still pending, this achievement marks a significant step forward in the field of AI-driven drug discovery.
Generative AI models are being used to design novel molecules with specific properties. These AI systems can propose drug candidates with optimized binding affinity, selectivity, and pharmacokinetic properties, streamlining the drug development process.
During the COVID-19 pandemic, AI played a crucial role in identifying potential treatments. Researchers used AI to screen existing drugs for their ability to inhibit the SARS-CoV-2 virus. This approach led to the discovery of several promising drug candidates, expediting the search for effective COVID-19 therapies.
While AI holds immense promise in drug discovery, it is not without its challenges and ethical considerations. Some of the key issues include:
Data Quality: AI algorithms rely on high-quality, unbiased data. Ensuring the integrity and accuracy of the data used in drug discovery is essential to avoid erroneous predictions and potential harm to patients.
Bias in AI Algorithms: AI algorithms can inherit biases present in the data they are trained on. To ensure fairness and equity in drug development, efforts must be made to identify and mitigate algorithmic biases.
Regulatory Approval: Regulatory agencies, such as the FDA, are still adapting to the use of AI in drug development. Establishing clear guidelines for the validation and approval of AI-generated drugs is an ongoing challenge.
Privacy Concerns: The use of patient data for AI-driven personalized medicine raises privacy concerns. Striking a balance between patient data protection and medical advancements is a delicate ethical issue.
The future of AI in drug discovery looks promising, with continued advancements and breakthroughs on the horizon. Here are some key developments to watch for:
Drug Discovery Ecosystem: AI will become an integral part of the drug discovery ecosystem, working alongside human researchers to accelerate the process and increase success rates.
Drug Repurposing: AI-driven drug repurposing will continue to identify new uses for existing medications, potentially leading to cost-effective treatments for a wide range of diseases.
AI-Designed Drugs: AI-designed drugs will become more common, with algorithms creating innovative molecules tailored to specific therapeutic targets.
Personalized Medicine: Precision medicine will expand, with AI algorithms analyzing patient data to prescribe the most effective treatments with minimal side effects.
Regulatory Adaptation: Regulatory agencies will adapt to the use of AI in drug discovery, establishing guidelines and standards to ensure the safety and efficacy of AI-generated drugs.
AI's role in drug discovery is nothing short of revolutionary. By harnessing the power of machine learning, deep learning, and big data, AI is unlocking new possibilities in the pharmaceutical industry. From drug repurposing to personalized medicine and AI-designed molecules, the impact of AI is already evident in real-world applications.
However, it is crucial to address challenges related to data quality, bias, regulation, and ethics to ensure that AI continues to benefit patients and drive innovation in drug discovery. As we look to the future, the collaboration between AI and human researchers promises to bring about a new